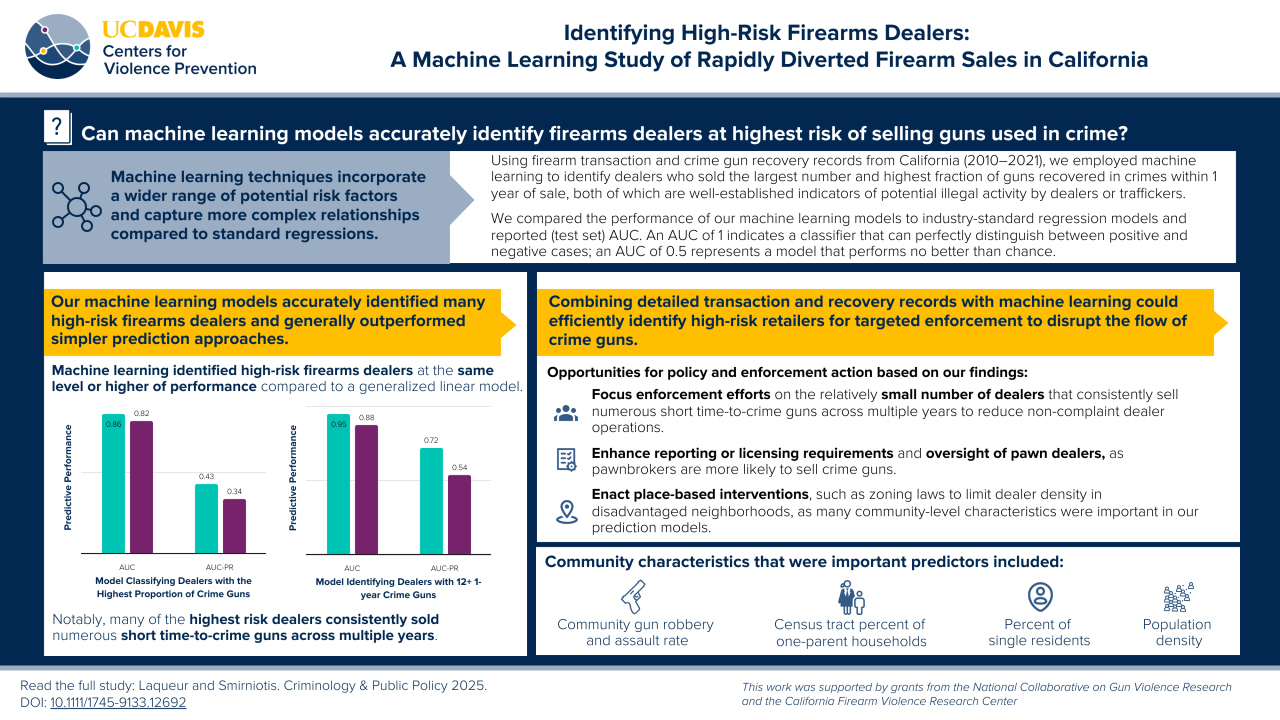
Identifying high-risk firearms dealers
A machine learning study of rapidly diverted firearm sales in California
Quick Summary
- Firearms dealers can facilitate the diversion of guns to the criminal market through practices such as selling to straw purchasers or failing to conduct required background checks.
Abstract
Research Summary
Using firearm transaction and crime gun recovery records from California (2010–2021), we employ machine learning to identify dealers who sold largest number and highest fraction of guns recovered in crimes within 1 year of sale. This short “time-to-crime” (TTC) is a well-established indicator of potential illegal activity by dealers or traffickers. We developed two primary prediction models: the first classifies dealer-years in the top 5% of 1-year crime gun sales volume (prediction model 1), the second identifies dealer-years in the top 5% based on the fraction of sales recovered within a year (prediction model 2). Both models demonstrated strong discriminative performance, with areas under the receiver operating curve (AUCs) of 0.95 and 0.86, respectively, and areas under the precision-recall curve (AUC–PRs) of 0.72 and 0.43. By comparison, a random classifier would be expected to achieve an AUC of 0.50 and an AUC-PR of 0.05. Prediction model 1 was particularly effective at identifying the highest risk dealers: Those with predictions exceeding 0.90 consistently ranked in the top 5% across multiple years, averaging 33 1-year crime gun sales annually. The machine learning models generally outperformed simpler regression and rule-based approaches, underscoring the value of data-adaptive methods for prediction. Key predictors included prior-year crime gun sales, the average age of purchasers, the proportion of “cheap” handgun sales, and the local gun robbery and assault rate.
Media Resources
- Read the study in Criminology & Public Policy
- View the visual abstract